How To Use Descriptive, Predictive and Prescriptive Analytics in Your Mobile App
Find out how predictive, prescriptive and descriptive analytics can be used to interpret the data of your app and help improve your app’s performance.
5 min. read - November 10, 2016
There’s no point in implementing mobile app analytics and measurement tools if you don’t intend to use the data gathered to optimize the app and improve its performance. If you’re going to spend your company’s time and resources instrumenting and measuring user behavior, you’d better have a plan for harvesting that data and turning it into actionable insight.
The first step is understanding the different ways you can use data. Although there are many different mobile analytics methodologies (and there seem to be more appearing every day), the three main types of analytics are descriptive, predictive, and prescriptive. You’ll probably use a combination of all three — though how to do it depends on your immediate objectives for the app and your business.
Descriptive analytics
Descriptive analytics is generally backwards looking, describing what actually happened versus what you expected to happen. Descriptive analytics measures facts and summarizes data to see if you achieved what you set out to do. Did you meet the targets you set in your measurement plan?
Old-fashioned reporting
A typical descriptive analysis starts with what we’ve always known as reporting. An analyst pulls data from a data repository — whether that’s an app store, a third-party analytics tool, or maybe even a custom dashboard — summarizes it, and compares it against targets and previous date ranges. The analyst not only uses subject matter expertise to make judgments about the data, but should also have clear targets in mind that indicate whether the app is achieving its goals. For example, the dashboard could show that the app is trending at around 500 users per day, and adding about 10 new users to that total each day. The analyst knows to concentrate on that trend because new users per day is the one metric that matters (OMTM) most. Any decline in that value is a red flag that should spur you to dig deeper into the data to find out what could be causing the fall, and identify actions to take that can reverse the decline.
It’s tempting to only look for successes in your analysis and gloss over problems indicated in the metrics. But you can derive real value from identifying what didn’t happen, where you didn’t meet the targets you were hoping to achieve, then trying to understand what went wrong. If you see a fall in the new users per day metric, your analysts could look for the cause using segmentation and cohort analysis.
Segmentation
A first step in any deeper dive is segmenting your audience into groups based on shared variables. Segmentation takes into account the fact that although each individual is different, they may share certain characteristics with others. Identifying those shared characteristics allows you to make decisions about what is happening with your app.
For example, is a fall in new sessions affecting segments equally? Did you see consistent sessions on iOS, but Android sessions dropped off a cliff? Or was it a particular demographic group, or sessions from a specific campaign or channel that saw a big dip?
When you know who — or more accurately, what group — is affecting the change in metrics, it is much easier to see why the change is taking place. What is particular to that group of users that they are no longer using the app? What do you need to fix to get back on track to meeting your targets?
Cohort analysis
Cohort analysis is closely related to segmentation. Rather than simply looking at a shared characteristic to create a segment, a cohort also features the shared dimension of time. For example, one cohort could be defined as every user who downloaded the app in January. The next cohort is users who downloaded in February, and so on. The time period of any cohort depends on your objectives, but you can track these users over time to see how often they return, their daily usage, or their average spend — whatever is important to your business.
The benefit of cohort analysis is that you have an apples-to-apples comparison of users over time. This will reveal whether the “quality” of your user base is improving (in the context of your goals).
Empower your whole team
Descriptive analytics is not only the domain of analysts and the finance team. If done well, standard reports and dashboards can be powerful self-service tools for your whole team. Your OMTM can be part of a shared dashboard — or even a widget that can be shared on individual dashboards that relate to specific team member’s goals.
Your data must be clear, meaningful, and targeted. Everyone who looks at the data should know exactly what they are looking at and why it matters. Whether you’re having a conversation with your CFO, a sales director or a customer service rep, you should all speak the same language.
Predictive analytics
Predictive analytics is the opposite of descriptive analytics, as it provides a way of looking into the future. Where can we expect the business to go given the situation today? Of course, no one has a crystal ball. However, predictive analytics isn’t just about looking forward. It is the process of predicting data you don’t have based on data you do have — and can be applied to help you make decisions based on what’s happening today. It involves identifying trends in the data (modeling) and extrapolating them, allowing you to plan and forecast.
For example, analysts could look at the growth of new users of the app and extrapolate that trend to help plan next month’s ad spend. Or they could look at recent user behavior and identify what might make it more likely for users to make in-app purchases. Perhaps the top revenue generators are in a particular demographic group, or are heavy users of the app, or open the app only on weekends, or share any other variable you can identify. Often, these characteristics are given a weighted score in a model that analysts can then use to decide the level of resources to apply to improve results.
Analysts can also use predictive analytics to explore different tactics that will grow the business or increase the app’s success. Developing hypotheses this way and testing them in the real world is an element of the third approach, prescriptive analytics.
Prescriptive analytics
Prescriptive analytics is sometimes thought of as a subset of predictive analytics, mainly because it’s also forward looking. Insight gained from prescriptive analytics can help inform decisions and answer questions like, “What should we do next? How can we improve? What actions does the data indicate that will help us achieve higher KPI targets — or perhaps that we should switch tactics?”
Prescriptive analytics should be an essential part of your strategic decision making. Essentially, it helps you answer the “how do we get there?” question that follows the predictive analytics “where do we want to go?” question.
You want to identify the particular metric to improve (descriptive), model how it could change (predictive), then create scenarios that will improve it from the expected values you obtain from the model (prescriptive). Doing this gives you metrics-based, testable hypotheses that will inform changes you need to make to improve your app.
Derive and test hypotheses
Testing your hypotheses can be as simple or complex as you choose, and may include:
Standard A/B tests
More complicated multivariate tests
Longitudinal tests where you compare time periods
Longitudinal tests can be tricky because you can’t control for all variables. The point is you don’t need to guess. You’ll gather information to tell you whether your hypothesis is correct or not.
To be successful, you’ll need three elements:
A testable hypothesis that the data is able to disprove (for example, “the green button will be clicked at a greater rate than the red button,” not “people like green better than red”)
Actionable data (of course)
A feedback mechanism that lets you analyze the results of your test and take further action
The feedback mechanism is crucial because your results should allow you to refine the objectives and tactics in your measurement plan. Even if your hypothesis was incorrect, you now have data that will help you change your tactic, set an objective, model it, and test a new hypothesis.
Free ebook on mobile app analytics
To learn more, download our free mobile app analytics ebook: The Smart App: A Comprehensive Guide for Using Mobile Analytics to Make Your App a Success — Right from the Start.
Article Author:
Subscribe for more insights
Get our newsletter in your inbox.
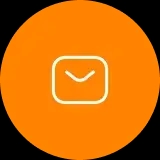
Contact us.
Let's build something lovable. Together.
We help companies of all sizes build lovable apps, websites, and connected experiences.