The Potential of ResearchKit: Q&A with Dr. Samuel Blackman
Learn how one doctor, who designs clinical trials for new cancer medications, thinks ResearchKit and mobile technology may revolutionize how he works.
12 min. read - March 26, 2015
Ed’s Note: The opinions expressed by Dr. Samuel Blackman in this blog post are his own, and do not reflect the opinions of his employer.
For the past seven years, Dr. Samuel Blackman has been trying to help cure cancer. Literally.
The Seattle resident designs clinical trials for pharmaceutical companies, testing the effects of new cellular therapies on cancer patients.
When Apple announced ResearchKit at its recent press conference, it caught Blackman’s attention. He’s been thinking about how mobile technology could revolutionize his job and his industry for years.
“When I finished my clinical training, my hospital was just moving to electronic records and the term ‘big data’ hadn’t been coined,” he told me during a recent video chat. “But even then we started thinking about how all the data and analytics might really change medical practice.”
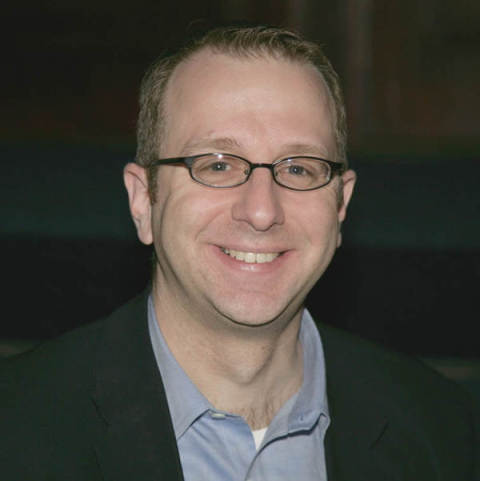
DR. SAMUEL BLACKMAN - Senior Vice President / Silverback Therapeutics
Fast forward to today, and Blackman says one of the fundamental issues with cancer drug development is the disconnect between the measurable outcomes and how the patient is really doing as a whole person.
“The outcomes we measure and track are pretty basic — you are either in remission or you aren’t, or the tumor might be getting larger or smaller,” he says. “But from a patient point of view, you could have a tumor that isn’t shrinking, but you could feel much better.”
Blackman sees a not-too-distant future where clinical trials will include a wealth of new data, thanks to the technology built into phones and other wearables in development, along with tools like Apple’s ResearchKit that make developing apps and capturing data from hardware easier.
Tracking patient vital signs, movements, eating, and sleeping habits — and even using push notifications to probe those patients for subjective information like their current pain levels or their mood — will change how studies are conducted, Blackman says. With this richer dataset, Blackman thinks that our understanding of the success (or failure) of certain trials will be deeper, and that decisions from early stage trials as to whether a new drug is likely to succeed or not could be made more quickly and affordably.
Here’s much more from my conversation with Blackman:
When did you first start thinking about using mobile devices to help with medical research?
Three years ago, I bought a Fitbit because it was cool and new and I’ve always been an early adopter. After wearing it for while and seeing the data come from it, I realized it could provide me with some valuable information beyond just the number of steps I took. On the days I was sick, I noticed my activity level would go way down and I wasn’t eating as much and my sleep quality wasn’t as good. When I felt better, those changes would be reversed. You could start to see clear patterns over time.
So, I started to think about the cancer patients involved in the clinical trials that I was running. The per-patient budget for some of these trials is $10,000 or more, so $300 for a Fitbit and an iPhone as a data-gathering tool would be a marginal cost. As the treatment is implemented, you could monitor the objective data provided by Fitbit, like how much patients move around and whether they are becoming more active as the treatment proceeds. We could even push subjective questions out to them, asking how good they feel today or how they would rate their pain or energy on a scale.
“My suspicion is that most people are willing to sacrifice some degree of privacy for either a purpose they believe in or for some reasonable return.”
Today, the big cancer drug experiments can cost tens or hundreds of millions of dollars and take up to five years to determine if there’s a survival benefit. There’s also a huge opportunity cost in these large experiments. If we spend five years on one project and it takes that long to find out it’s not successful, then what projects didn’t I spend that time or money on that might have been successful? Tools like iPhone and ResearchKit could help drug developers more efficiently identify new drugs that have a higher probability of success and weed out those that don’t in Phase 1 or Phase 2 trials — before things get expensive.
There’s a lot of concern about privacy and sharing personal medical information. It seems like as a society we’re becoming more comfortable with digitally sharing some kinds of personal information. But how big of a challenge will privacy be for ResearchKit?
Privacy is one of the foundations for the Hippocratic Oath, so the sensitivity about personal information in medicine goes back thousands of years. But today we are being tracked by the web pages we visit, and even by government agencies. In a way, the notion of electronic privacy is an illusion. So, I don’t know if concerns about sharing biometric data from these devices will remain as high in the long term.
My suspicion is that most people are willing to sacrifice some degree of privacy for either a purpose they believe in or for some reasonable return. Almost all of us agree to our Fitbit and iTunes end-user licenses without reading them. I don’t know what Fitbit does with my data on the back-end but I’m sure they are doing some type of large analytics. But I get something in return for this “loss of privacy” — these fantastic individual analytics about my activity I can use for my own health monitoring. Hundreds of thousands of people signed up for 23andme and shared information about their DNA. That’s a big deal — it indicates that people are willing to share very intimate data to help solve larger healthcare problems. How that translates as we ask people to share more direct information about their health conditions, I don’t know — but it will be interesting to see.
In the drug development space, it’s a little bit different. Many of the patients enrolled in cancer clinical trials are desperately ill and have exhausted all standards of care. In return for participating in clinical trials, they gain access to new therapies. And if successful, we can apply those therapies to a broader population. I’ve found most patients on clinical trials are very willing to share data if they know it will help — even if it won’t help them directly, but will help our understanding of their disease or the new therapy under development. I believe that most patients, when it’s explained to them, see the bigger picture.
What are some exciting/important use cases for ResearchKit that Apple hasn’t mentioned?
I could spend all day riffing on this. But let me give you a few.
We are reaching, if we’ve not reached or exceeded, our capacity for healthcare costs in society. So at some point, the people who are paying the bill for healthcare — taxpayers, insurers, employers, etc. — are going to ask even more loudly how we are getting value out of the money we’re spending. And it’s a really hard question to answer. Identifying and building tools that demonstrate ROI using these programs could be incredibly valuable to payers. And if you can use electronic tools to more easily capture how treatments affect patients, that makes it much easier to justify the cost to the system. It also makes drug development resource decisions much easier.
Another use case is establishing a normal population data set. When you run clinical trials, you are trying to measure the effect of your drug on a population. For example, if I develop an arthritis pill and a patient shows some improvement, that’s great. But how does that compare to normal? With electronic tools like iPhone and ResearchKit, you could easily gather very large baseline datasets on a matched normal population for very little money. You probably don’t think normal data sounds sexy — but from a research point of view, it’s immensely valuable.
The other case I often think about is patients that are at risk for some type of medical catastrophe or hospitalization. When you are under anesthesia, you are intensely monitored. Your heart rate, blood pressure, the amount of oxygen in your blood, the carbon dioxide levels in your breath — physicians are watching changes in those variables to help avoid catastrophic events, like a heart attack. With these technologies, you could have similar monitoring taking place all the time for patients after surgery, either in the hospital or at home.
“This is medical care the way it should be — an extension of the healthcare system right to the patient’s bedside, wherever they are.”
Here’s one example: Let’s say an elderly patient is recovering from hip replacement. When she’s discharged and at home, you could monitor her remotely for activity levels and symptoms that predict a good outcome or a bad outcome. If she’s stuck in bed due to inadequate pain management, she might have a hard time getting up to get water. So she starts to get dehydrated. And if she gets dehydrated, she might get a blood clot, and if she gets a blood clot, she may get a pulmonary embolism — and she’ll wind up back in the hospital. The root cause is that her pain management was inadequate so she couldn’t mobilize herself, which is a key part of recovery. To intervene, you could ping her iPhone asking her how many glasses of water she has had. Or, if you see her vitals change, or the day-to-day change in the number of steps she’s taken, and if she hasn’t moved much, you could automatically dispatch a home-health nurse, or call her family to check on her. That could save the healthcare system an immense amount of money — and save that patient an immense amount of discomfort.
This is medical care the way it should be — an extension of the healthcare system right to the patient’s bedside, wherever they are.
Is the instrumentation in the iPhone and other devices really good enough for some of the ResearchKit use cases people are talking about?
It’s an important question. There was a recent paper from the FDA about mobile medical apps and whether or not they have been adequately validated. There are definitely some data integrity issues industry is going to need to figure out. But my suspicion is that there are technological fixes for any issues. We’re pretty good at isolating data that may be bad. For example, if you take your iPhone and wiggle it around for an hour, there’s no normal behaviors in life that will look like that. You can begin to remove questionable patterns through vigilant pattern recognition.
What do you see as the biggest stumbling blocks to the adoption of ResearchKit?
Data integrity is going to be important, for everything, but especially in drug development. We spend an enormous amount of effort making sure the data we capture truly proves our drugs are going to work. For every data point that a physician puts into our system for a clinical trial, we send people out to the site to verify that data point in the primary source document: the physical medical record. We need to know the foundation of the data is solid before we submit our data to the FDA. This will need to be addressed for the new devices.
Defining normal is also going to be important. We could rush out and gather data on 100,000 sick people, but unless you have a normal set for comparison, it will be hard to interpret the data and come to any conclusions. It’s incredibly important to understand baseline data in order to understand what is improvement and what is detriment.
“What if 23andMe started sending me ads about remedies for hair loss because they knew about my male-pattern baldness gene? I’d be pretty irritated.”
Last, we have to avoid using data like this for marketing. Most people have learned to live with ads on Google based on their searches and what’s in their inbox. For companies closer to healthcare, there may be temptation to exploit this new data for commercial purposes. Let’s say you wanted build a healthcare app to gather data to correlate weather with mood and activity. You are using iPhone and Fitbit data to determine whether people in Seattle exercise less or are are more moody because of the weather (we don’t, and we’re not, by the way). That kind of data could theoretically be very interesting to the manufacturer of an antidepressant. I suspect that many people would balk if they started getting advertisements for anti-depressants based on their Fitbit data. What if 23andMe started sending me ads about remedies for hair loss because they knew about my male-pattern baldness gene? I’d be pretty irritated.
We also need to make sure that privacy extends in a way that people don’t get penalized for certain behaviors. For example, what if you don’t exercise and you’re slightly overweight, and this data is tracked by your insurance company via a health improvement app they offer their subscribers. Does that open the door for insurance companies to raise premiums for those who are less fit? Should that be something we want to do as a society?
These tools will invariably open the door for a lot of other social, political, economic, ethical, and philosophical questions. That’s why it’s such an exciting field and time. What’s happening with mobile technology and tools like ResearchKit could have an enormous impact on medicine and healthcare. I’d really encourage your readers to think broadly about the potential of these new technologies, but also of the risks and pitfalls.
Article Author:
Subscribe for more insights
Get our newsletter in your inbox.
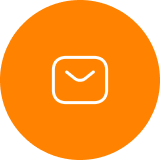
Contact us.
Let's build something lovable. Together.
We help companies of all sizes build lovable apps, websites, and connected experiences.